Graph Neural Network
The goal of this project is to develope a model that learns to map from parcel geometry to building footprint geometry
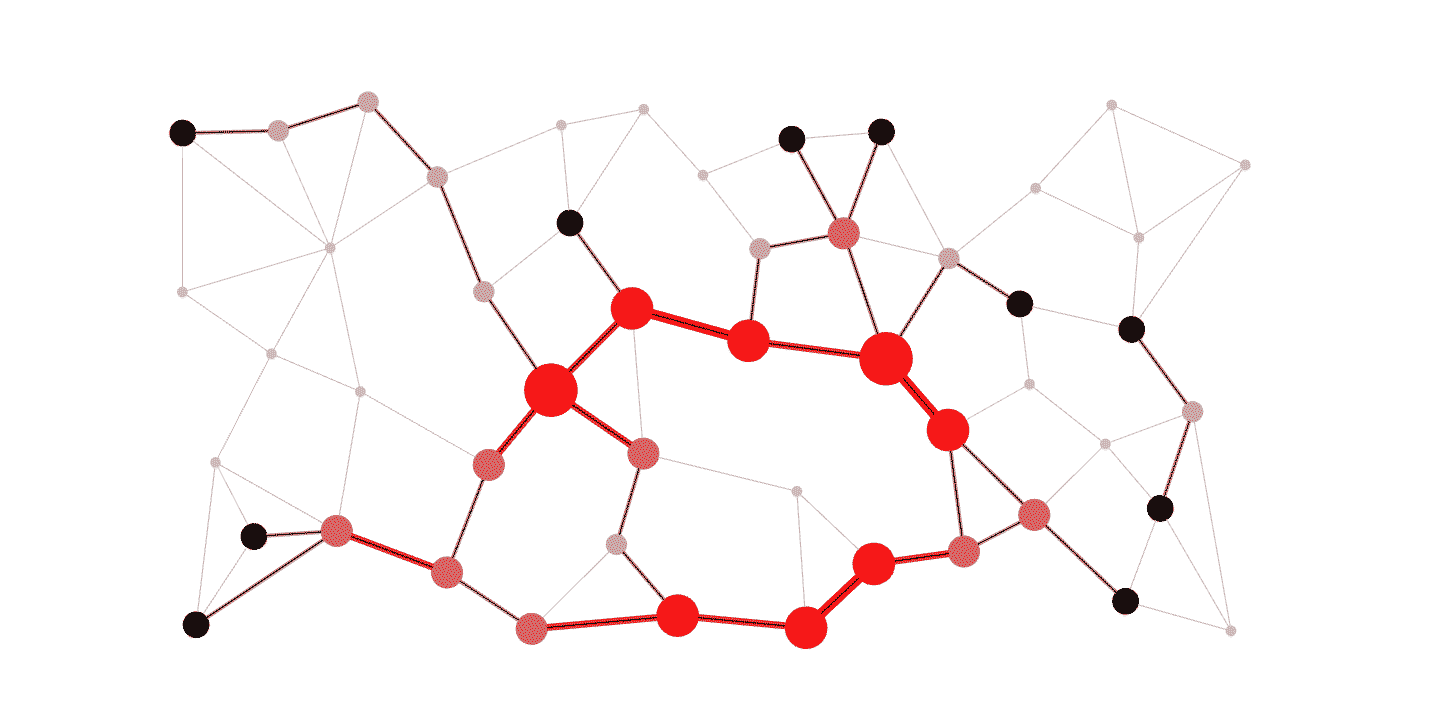
Graph Net-based Recommender Systems & Success of Graph Nets in drug development domain
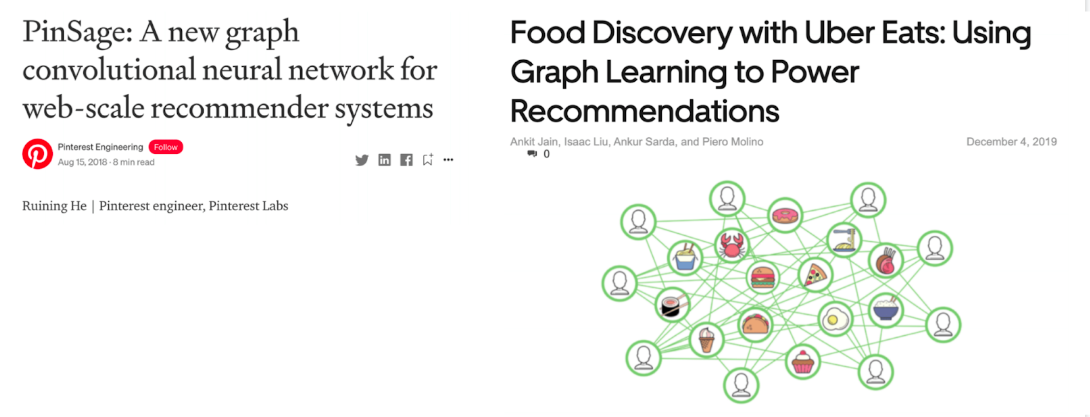
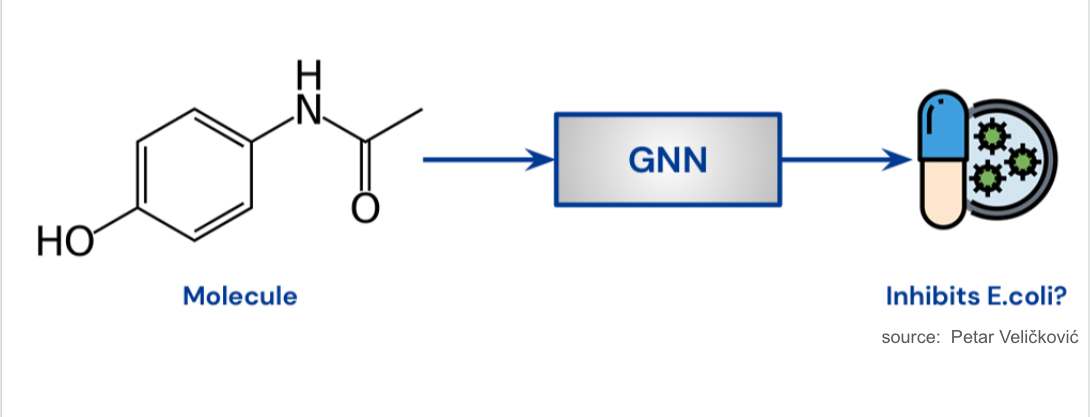
1. Originally designed for machine translation, but tons of state-of-the-art use cases even beyond NLP (e.g. vision transformers)
2. Excel at sequence-to-sequence (seq2seq) learning, and have largely replaced RNNs/LSTM for this use case
3. Transformers are attentional graph neural networks on a fully connected graph
4. Example: Transformer on SMILES for molecular drug discovery "SMILES expresses the structure of a given molecule in the form of an ASCII string” Train a seq2seq auto-encoder. Then use to generate new molecules.
Building footprint generation as seq2seq
String representation of geometries
1. Parcel: POLYGON ((102 14, 80 11, 37 0, 0 114, 89 127, 102 14))
2. Building: POLYGON ((94 34, 38 22, 25 85, 80 96, 94 34))
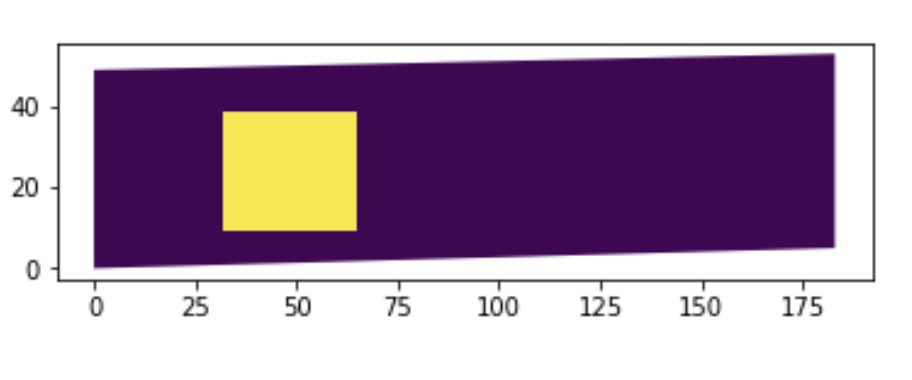
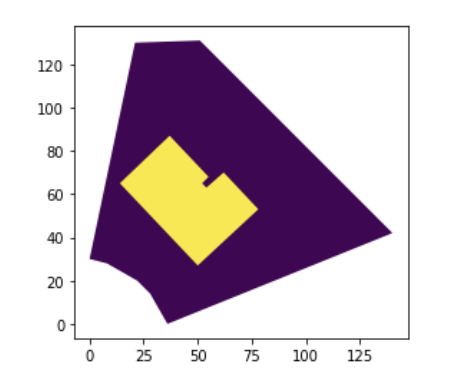
Numerical encoding of parcels and buildings
1. Parcel: 000000000049183053183005
2. Building: 032009032039065039065009
Encoded CMAP parcel geometries and building footprint geometries. Selected 41000 simple single-family building examples for initial prototype. 90% for train, 10% for test.
Transformer model to map parcel geometry to building geometry
Numeric representation of parcel geometry as a sequence of tokens
Convert the sequence of tokens to a one-hot integer representation
Encoder: Encode the discrete representation of a geometry into a real-valued continuous vector
Decoder: Convert continuous vector back to discrete geometry representation of the building geometry
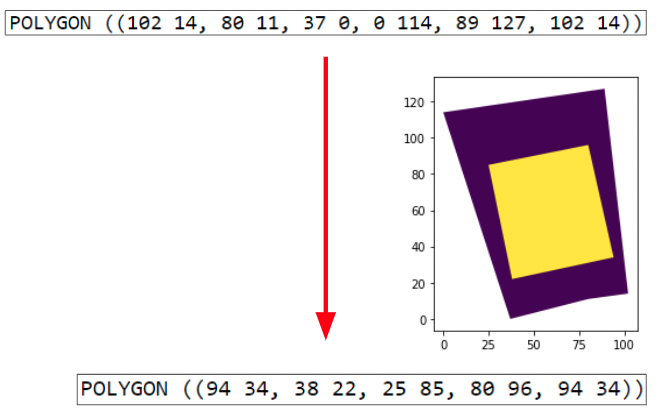
Transformers are GNNs on a fully connected graph
Transformers Test Examples
Why this is Interseting?
1. Can generate geometries of arbitrary length (as input and output). So should be able to handle e.g. complex multi-building footprints and very weird parcel shapes.
2. Use as input to other differentiable models. E.g. train a model of energy efficiency on the transformer latents. Then backprop from the energy efficiency model back to the latents to do “inverse design” of energy-efficient buildings.
3. Is differentiable and can connect to other differentiable models
4. Heuristic/combinatorially-optimized model -> Model learned from data
5. Connect with other differentiable models GNN -> Buildformer: Generated building as a function of GNN embeddings for each submarket, as well as site characteristics (allowable height, number of structures, which side is frontage, estimated profitability, year built)